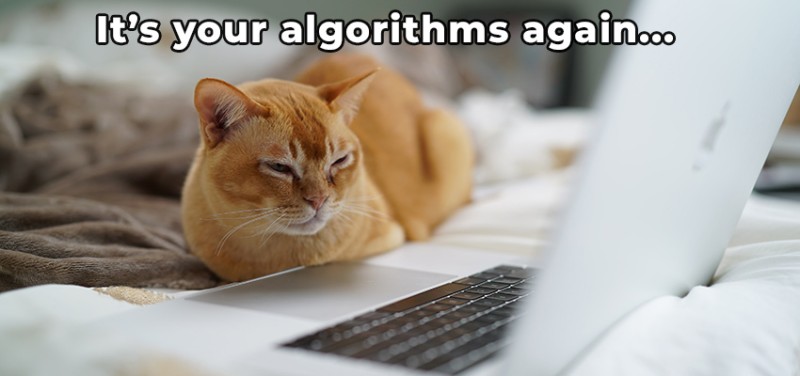
- V è un insieme di vertici;
- R è un insieme di linee che collegano coppie di vertici.
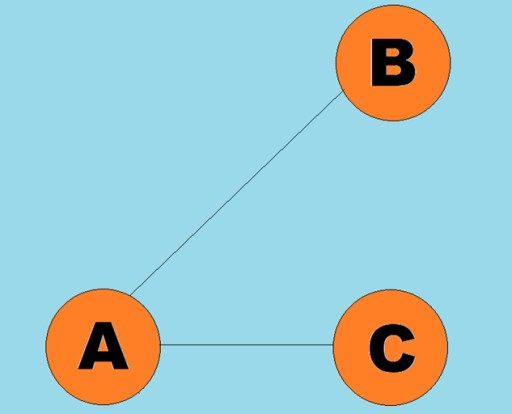
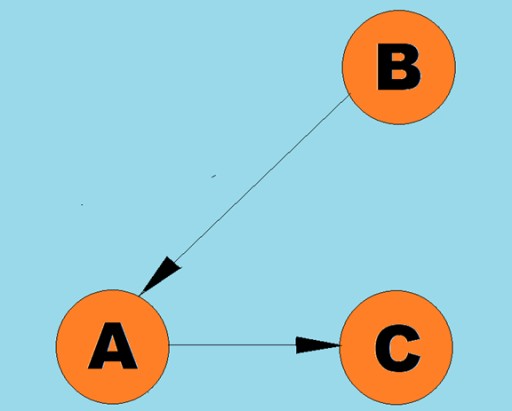
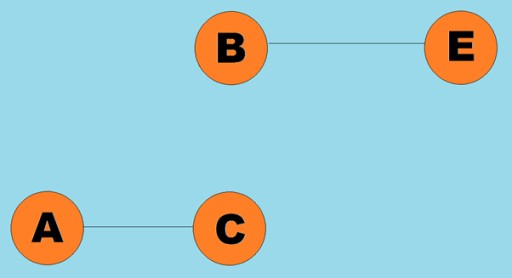
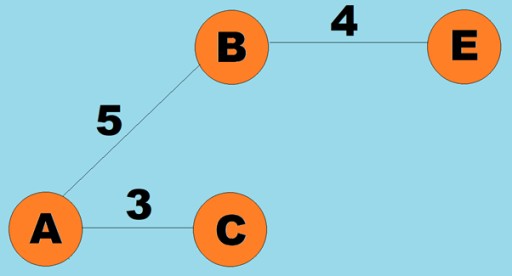
3. Algoritmi di pathfinding (profondità prima, ampiezza prima)
Una delle operazioni fondamentali eseguite con i grafici è determinare tutti i vertici raggiungibili da un dato vertice. Immagina di provare a determinare come viaggiare in autobus da una città all'altra, compresi i possibili trasferimenti. Alcune città possono essere raggiunte direttamente, mentre altre possono essere raggiunte solo passando per altre città. Esistono molte altre situazioni in cui potresti dover trovare tutti i vertici che puoi raggiungere da un dato vertice. Esistono due modi principali per attraversare i grafici: prima in profondità e prima in ampiezza. Esploreremo entrambi questi aspetti. Entrambi i metodi attraversano tutti i vertici connessi. Per esplorare ulteriormente gli algoritmi deep-first e width-first, utilizzeremo il seguente grafico: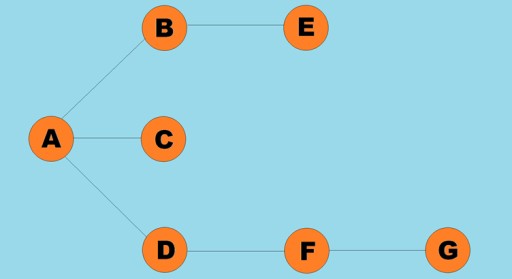
Ricerca in profondità
Questo è uno dei metodi di attraversamento del grafico più comuni. La strategia deep-first consiste nell'andare il più in profondità possibile in un grafico. Quindi, dopo aver raggiunto un vicolo cieco, torniamo al vertice più vicino che ha vertici adiacenti precedentemente non visitati. Questo algoritmo memorizza le informazioni nello stack su dove tornare quando viene raggiunto un vicolo cieco. Regole per la ricerca approfondita:- Visita un vertice adiacente precedentemente non visitato, contrassegnalo come visitato e mettilo nello stack.
- Spostarsi su questo vertice.
- Ripetere il passaggio 1.
- Se il passo 1 è impossibile, torna al vertice precedente e prova a eseguire il passo 1. Se ciò non è possibile, torna al vertice precedente e così via, finché non troviamo un vertice dal quale possiamo continuare la traversata.
- Continua finché tutti i vertici non sono in pila.
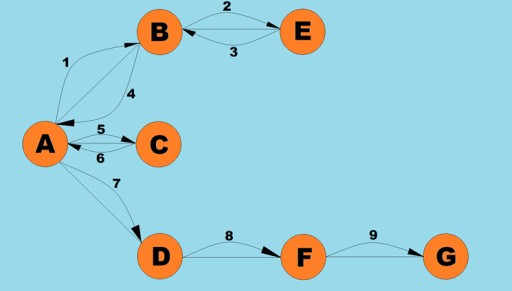
public class Graph {
private final int MAX_VERTS = 10;
private Vertex vertexArray[]; // Array of vertices
private int adjMat[][]; // Adjacency matrix
private int nVerts; // Current number of vertices
private Stack stack;
public Graph() { // Initialize internal fields
vertexArray = new Vertex[MAX_VERTS];
adjMat = new int[MAX_VERTS][MAX_VERTS];
nVerts = 0;
for (int j = 0; j < MAX_VERTS; j++) {
for (int k = 0; k < MAX_VERTS; k++) {
adjMat[j][k] = 0;
}
}
stack = new Stack<>();
}
public void addVertex(char lab) {
vertexArray[nVerts++] = new Vertex(lab);
}
public void addEdge(int start, int end) {
adjMat[start][end] = 1;
adjMat[end][start] = 1;
}
public void displayVertex(int v) {
System.out.println(vertexArray[v].getLabel());
}
public void dfs() { // Depth-first search
vertexArray[0].setVisited(true); // Take the first vertex
displayVertex(0);
stack.push(0);
while (!stack.empty()) {
int v = getAdjUnvisitedVertex(stack.peek()); // Return the index of the adjacent vertex: if any, 1; if not, -1
if (v == -1) { // If there is no unvisited adjacent vertex
stack.pop(); // The element is removed from the stack
}
else {
vertexArray[v].setVisited(true);
displayVertex(v);
stack.push(v); // The element goes on top of the stack
}
}
for (int j = 0; j < nVerts; j++) { // Reset the flags
vertexArray[j].visited = false;
}
}
private int getAdjUnvisitedVertex(int v) {
for (int j = 0; j < nVerts; j++) {
if (adjMat[v][j] == 1 && vertexArray[j].visited == false) {
return j; // Returns the first vertex found
}
}
return -1;
}
}
Un vertice assomiglia a questo:
public class Vertex {
private char label; // for example, 'A'
public boolean visited;
public Vertex(final char label) {
this.label = label;
visited = false;
}
public char getLabel() {
return this.label;
}
public boolean isVisited() {
return this.visited;
}
public void setVisited(final boolean visited) {
this.visited = visited;
}
}
Eseguiamo questo algoritmo con vertici specifici e vediamo se funziona correttamente:
public class Solution {
public static void main(String[] args) {
Graph graph = new Graph();
graph.addVertex('A'); //0
graph.addVertex('B'); //1
graph.addVertex('C'); //2
graph.addVertex('D'); //3
graph.addVertex('E'); //4
graph.addVertex('F'); //5
graph.addVertex('G'); //6
graph.addEdge(0,1);
graph.addEdge(0,2);
graph.addEdge(0,3);
graph.addEdge(1,4);
graph.addEdge(3,5);
graph.addEdge(5,6);
System.out.println("Visits: ");
graph.dfs();
}
}
Uscita console:
Ricerca in ampiezza
Come la ricerca approfondita, questo algoritmo è uno dei metodi di attraversamento del grafico più semplici e basilari. L'essenza è che abbiamo qualche vertice attuale. Mettiamo in coda tutti i suoi vertici adiacenti non visitati e selezioniamo l'elemento successivo (che è il vertice memorizzato in testa alla coda), che diventa il vertice corrente… Suddividendo questo algoritmo in fasi, possiamo identificare i seguenti passaggi:- Visita il successivo vertice precedentemente non visitato adiacente al vertice corrente, contrassegnalo in anticipo come visitato e aggiungilo alla coda.
- Se il passaggio n. 1 non può essere eseguito, rimuovere il vertice dalla coda e renderlo il vertice corrente.
- Se i passaggi n. 1 e n. 2 non possono essere eseguiti, l'attraversamento è terminato: ogni vertice è stato attraversato (se abbiamo un grafico connesso).
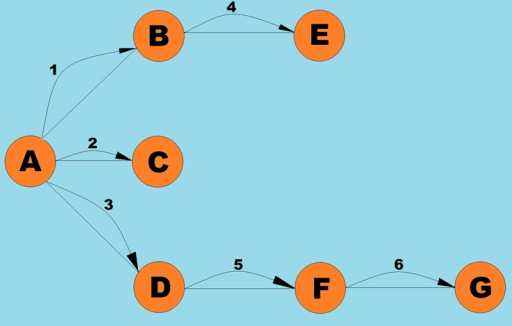
public class Graph {
private final int MAX_VERTS = 10;
private Vertex vertexList[]; // Array of vertices
private int adjMat[][]; // Adjacency matrix
private int nVerts; // Current number of vertices
private Queue queue;
public Graph() {
vertexList = new Vertex[MAX_VERTS];
adjMat = new int[MAX_VERTS][MAX_VERTS];
nVerts = 0;
for (int j = 0; j < MAX_VERTS; j++) {
for (int k = 0; k < MAX_VERTS; k++) { // Fill the adjacency matrix with zeros
adjMat[j][k] = 0;
}
}
queue = new PriorityQueue<>();
}
public void addVertex(char lab) {
vertexList[nVerts++] = new Vertex(lab);
}
public void addEdge(int start, int end) {
adjMat[start][end] = 1;
adjMat[end][start] = 1;
}
public void displayVertex(int v) {
System.out.println(vertexList[v].getLabel());
}
public void bfc() { // Depth-first search
vertexList[0].setVisited(true);
displayVertex(0);
queue.add(0);
int v2;
while (!queue.isEmpty()) {
int v = queue.remove();
while((v2 = getAdjUnvisitedVertex(v))!=-1) {// The loop runs until every adjacent vertex is found and added to the queue
vertexList[v2].visited = true;
displayVertex(v2);
queue.add(v2);
}
}
for (int j = 0; j < nVerts; j++) { // Reset the flags
vertexList[j].visited = false;
}
}
private int getAdjUnvisitedVertex(int v) {
for (int j = 0; j < nVerts; j++) {
if (adjMat[v][j] == 1 && vertexList[j].visited == false) {
return j; // Returns the first vertext found
}
}
return -1;
}
}
La classe Vertex è identica a quella che abbiamo utilizzato con l'algoritmo di ricerca deep-first. Mettiamo in azione questo algoritmo:
public class Solution {
public static void main(String[] args) {
Graph graph = new Graph();
graph.addVertex('A'); //0
graph.addVertex('B'); //1
graph.addVertex('C'); //2
graph.addVertex('D'); //3
graph.addVertex('E'); //4
graph.addVertex('F'); //5
graph.addVertex('G'); //6
graph.addEdge(0,1);
graph.addEdge(0,2);
graph.addEdge(0,3);
graph.addEdge(1,4);
graph.addEdge(3,5);
graph.addEdge(5,6);
System.out.println("Visits: ");
graph.bfc();
}
}
Uscita console:
4. Algoritmo di Dijkstra
Come accennato in precedenza, i grafici possono essere diretti o non orientati. E ricorderai che possono anche essere ponderati. I grafici ponderati e diretti modellano le relazioni che spesso si trovano nella vita reale: ad esempio, una mappa di città in cui le città sono i vertici e i bordi tra loro sono strade con traffico a senso unico, che scorrono nella direzione del bordo diretto. Diciamo che sei un'azienda di trasporti e devi trovare il percorso più breve tra due città distanti. Come lo faresti? Trovare il percorso più breve tra due vertici è uno dei problemi più comuni risolti utilizzando i grafici pesati. Per risolvere questo problema utilizziamo l'algoritmo di Dijkstra. Una volta eseguito, conoscerai i percorsi più brevi da un dato vertice iniziale a tutti gli altri. Quali sono i passaggi di questo algoritmo? Proverò a rispondere a questa domanda. Passi dell'algoritmo di Dijkstra:- Passaggio 1: trovare il nodo adiacente che ha il costo più basso verso cui navigare (peso del bordo più basso). Ti trovi proprio all'inizio e stai pensando a dove andare: al nodo A o al nodo B. Qual è il costo per spostarsi verso ciascuno di questi nodi?
- Passaggio 2: calcolare la distanza da percorrere verso tutti i vicini del nodo В che non sono stati ancora visitati dall'algoritmo quando si attraversa il bordo da В. Se la nuova distanza è inferiore a quella vecchia, allora il percorso attraverso il bordo B diventa il nuovo percorso più breve per questo vertice.
- Passaggio 3: contrassegnare il vertice B come visitato.
- Passaggio 4: vai al passaggio 1.
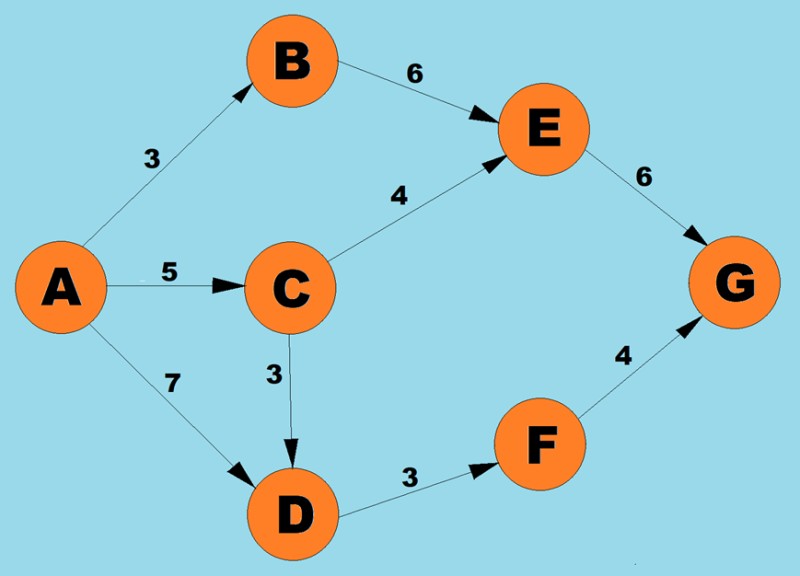
- Ci sono tre possibili percorsi per il vertice A: verso B con peso 3, verso С con peso 5 e verso D con peso 7. Secondo il primo passo dell'algoritmo, selezioniamo il nodo con il costo più basso (peso del bordo). In questo caso, B.
-
Dato che l'unico vicino non visitato di B è il vertice Å, controlliamo quale sarebbe il percorso se passassimo per questo vertice. 3(AB) + 6(BE) = 9.
Pertanto, registriamo che l'attuale percorso più breve da A a E è 9.
-
Poiché il nostro lavoro con il vertice B è completo, procediamo alla selezione del vertice successivo il cui bordo ha il peso minimo.
Dai vertici A e B, le possibilità sono i vertici D (7), C (5) o E (6).
Il bordo verso С ha il peso più piccolo, quindi andiamo su questo vertice.
-
Successivamente, come prima, troviamo il percorso più breve verso i vertici vicini quando si passa attraverso C:
-
AD = 5 (AC) + 3 (CD) = 8, ma poiché il percorso più breve precedente (AC = 7) è inferiore a questo attraverso С, manteniamo invariato il percorso più breve (AC = 7).
-
CE = 5(AC) + 4(CE) = 9. Questo nuovo cammino minimo è uguale al precedente, quindi lo lasciamo invariato.
-
-
Dai vertici accessibili più vicini (E e D), seleziona il vertice con il peso del bordo più piccolo, cioè D (3).
-
Troviamo il percorso più breve verso il suo vicino F.
AF = 7(AD) + 3(DF) = 9
-
Dai vertici accessibili più vicini (E e F), seleziona il vertice con il peso del bordo più piccolo, cioè F (3).
-
Trova il percorso più breve verso il suo vicino G.
AG = 7(AD) + 3(DF) + 4(FG) = 14
Quindi, abbiamo trovato un percorso da A a G.
Ma per essere sicuri che sia il più corto dobbiamo eseguire i nostri passi anche sul vertice E.
-
Poiché il vertice G non ha vertici vicini puntati da bordi diretti, ci rimane solo il vertice E, quindi lo scegliamo.
-
Trova il percorso più breve per il vicino G.
AG = 3 (AB) + 6 (BE) + 6 (EG) = 15. Questo percorso è più lungo del percorso più breve precedente (AG (14)), quindi lasciamo questo percorso invariato.
Poiché non ci sono vertici che partono da G, non ha senso eseguire i passaggi dell'algoritmo su questo vertice. Ciò significa che il lavoro dell’algoritmo è completo.
public class Vertex {
private char label;
private boolean isInTree;
public Vertex(char label) {
this.label = label;
this.isInTree = false;
}
public char getLabel() {
return label;
}
public void setLabel(char label) {
this.label = label;
}
public boolean isInTree() {
return isInTree;
}
public void setInTree(boolean inTree) {
isInTree = inTree;
}
}
La classe dei vertici è praticamente identica alla classe dei vertici utilizzata per la ricerca in ampiezza. Per visualizzare i percorsi più brevi, abbiamo bisogno di una nuova classe che conterrà i dati di cui abbiamo bisogno:
public class Path { // A class that contains the distance and the previous and traversed vertices
private int distance; // Current distance from the starting vertex
private List parentVertices; // Current parent vertex
public Path(int distance) {
this.distance = distance;
this.parentVertices = new ArrayList<>();
}
public int getDistance() {
return distance;
}
public void setDistance(int distance) {
this.distance = distance;
}
public List getParentVertices() {
return parentVertices;
}
public void setParentVertices(List parentVertices) {
this.parentVertices = parentVertices;
}
}
In questa classe possiamo vedere la distanza totale del percorso e i vertici che verranno attraversati passando lungo il percorso più breve. E ora diamo un'occhiata alla classe in cui effettivamente percorriamo il percorso più breve nel grafico. Quindi qui abbiamo la classe Graph:
public class Graph {
private final int MAX_VERTS = 10;// Maximum number of vertices
private final int INFINITY = 100000000; // This number represents infinity
private Vertex vertexList[]; // List of vertices
private int relationMatrix[][]; // Matrix of edges between vertices
private int numberOfVertices; // Current number of vertices
private int numberOfVerticesInTree; // Number of visited vertices in the tree
private List shortestPaths; // List of shortest paths
private int currentVertex; // Current vertex
private int startToCurrent; // Distance to currentVertex
public Graph() {
vertexList = new Vertex[MAX_VERTS]; // Adjacency matrix
relationMatrix = new int[MAX_VERTS][MAX_VERTS];
numberOfVertices = 0;
numberOfVerticesInTree = 0;
for (int i = 0; i < MAX_VERTS; i++) { // Fill the adjacency matrix
for (int k = 0; k < MAX_VERTS; k++) { // with "infinity"
relationMatrix[i][k] = INFINITY; // Assign default values
shortestPaths = new ArrayList<>(); // Assign an empty list
}
}
}
public void addVertex(char lab) {// Assign new vertices
vertexList[numberOfVertices++] = new Vertex(lab);
}
public void addEdge(int start, int end, int weight) {
relationMatrix[start][end] = weight; // Set weighted edges between vertices
}
public void path() { // Choose the shortest path
// Set the initial vertex data
int startTree = 0; // Start from vertex 0
vertexList[startTree].setInTree(true); // Include the first element in the tree
numberOfVerticesInTree = 1;
// Fill out the shortest paths for vertices adjacent to the initial vertex
for (int i = 0; i < numberOfVertices; i++) {
int tempDist = relationMatrix[startTree][i];
Path path = new Path(tempDist);
path.getParentVertices().add(0);// The starting vertex will always be a parent vertex
shortestPaths.add(path);
}
// Until every vertex is in the tree
while (numberOfVerticesInTree < numberOfVertices) { // Do this until the number of of vertices in the tree equals the total number of vertices
int indexMin = getMin();// Get the index of the of the vertex with the smallest distance from the vertices not yet in the tree
int minDist = shortestPaths.get(indexMin).getDistance(); // Minimum distance to the vertices not yet in the tree
if (minDist == INFINITY) {
System.out.println("The graph has an unreachable vertex");
break; // If only unreachable vertices have not been visited, then we exit the loop
} else {
currentVertex = indexMin; // Set currentVert to the index of the current vertex
startToCurrent = shortestPaths.get(indexMin).getDistance(); // Set the distance to the current vertex
}
vertexList[currentVertex].setInTree(true); // Add the current vertex to the tree
numberOfVerticesInTree++; // Increase the count of vertices in the tree
updateShortestPaths(); // Update the list of shortest paths
}
displayPaths(); // Display the results on the console
}
public void clear() { // Clear the tree
numberOfVerticesInTree = 0;
for (int i = 0; i < numberOfVertices; i++) {
vertexList[i].setInTree(false);
}
}
private int getMin() {
int minDist = INFINITY; // The distance of the initial shortest path is taken to be infinite
int indexMin = 0;
for (int i = 1; i < numberOfVertices; i++) { // For each vertex
if (!vertexList[i].isInTree() && shortestPaths.get(i).getDistance() < minDist) { // If the vertex is not yet in the tree and the distance to the vertex is less than the current minimum
minDist = shortestPaths.get(i).getDistance(); // then update the minimum
indexMin = i; // Update the index of the vertex with the minimum distance
}
}
return indexMin; // Returns the index of the vertex with the smallest distance among those not yet in the tree
}
private void updateShortestPaths() {
int vertexIndex = 1; // The initial vertex is skipped
while (vertexIndex < numberOfVertices) { // Run over the columns
if (vertexList[vertexIndex].isInTree()) { // If the column vertex is already in the tree, then we skip it
vertexIndex++;
continue;
}
// Calculate the distance for one element sPath
// Get the edge from currentVert to column
int currentToFringe = relationMatrix[currentVertex][vertexIndex];
// Add up all the distances
int startToFringe = startToCurrent + currentToFringe;
// Determine the distance to the current vertexIndex
int shortPathDistance = shortestPaths.get(vertexIndex).getDistance();
// Compare the distance through currentVertex with the current distance in the vertex with index vertexIndex
if (startToFringe < shortPathDistance) { // If it is smaller, then the vertex at vertexIndex is assigned the new shortest path
List newParents = new ArrayList<>(shortestPaths.get(currentVertex).getParentVertices()); // Create a copy of the list of vertices of vertex currentVert's parents
newParents.add(currentVertex); // And add currentVertex to it as the previous vertex
shortestPaths.get(vertexIndex).setParentVertices(newParents); // Save the new path
shortestPaths.get(vertexIndex).setDistance(startToFringe); // Save the new distance
}
vertexIndex++;
}
}
private void displayPaths() { // A method for displaying the shortest paths on the screen
for (int i = 0; i < numberOfVertices; i++) {
System.out.print(vertexList[i].getLabel() + " = ");
if (shortestPaths.get(i).getDistance() == INFINITY) {
System.out.println("0");
} else {
String result = shortestPaths.get(i).getDistance() + " (";
List parents = shortestPaths.get(i).getParentVertices();
for (int j = 0; j < parents.size(); j++) {
result += vertexList[parents.get(j)].getLabel() + " -> ";
}
System.out.println(result + vertexList[i].getLabel() + ")");
}
}
}
}
Quindi questa è tutta la magia =) Ora diamo un'occhiata a questo algoritmo in azione:
public class Solution {
public static void main(String[] args) {
Graph graph = new Graph();
graph.addVertex('A');
graph.addVertex('B');
graph.addVertex('C');
graph.addVertex('D');
graph.addVertex('E');
graph.addVertex('F');
graph.addVertex('G');
graph.addEdge(0, 1, 3);
graph.addEdge(0, 2, 5);
graph.addEdge(0, 3, 7);
graph.addEdge(1, 4, 6);
graph.addEdge(2, 4, 4);
graph.addEdge(2, 3, 3);
graph.addEdge(3, 5, 3);
graph.addEdge(4, 6, 6);
graph.addEdge(5, 6, 4);
System.out.println("The following nodes form the shortest paths from node A:");
graph.path();
graph.clear();
}
}
E l'output della console è questo:
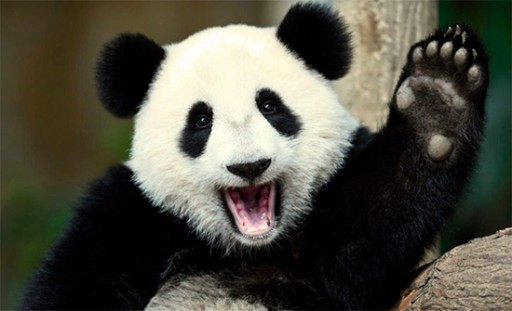
GO TO FULL VERSION